
In light of the #MeToo movement and with the growing push for transparency on equal pay and equal opportunity, the world of Tech has had to come to terms with its concerning lack of female representation. It is no secret that women make up a woeful proportion of employees in the tech workforce. Statista estimates the figure to be less than 1 in 4 actual tech roles are taken up by females. The figures are equally as bad, if not worse, for Data Science. According to a report by Harnham, in America, only 18% of data scientists are women and 11% of data teams have no women at all. However, the lack of gender representation (along with other forms of representation such as racial representation), specifically in Data and AI, has ramifications outside of the workplace. It can inhibit the pursuit of gender equality in society. Data and AI are doubly impacted by a lack of female representation due to the gender data gap that exists in much of our data, as highlighted by Caroline Criado-Perez. Representation of females in the data and in the design of data solutions should be essential in any business process.
Bias in Data
All Data Science and AI projects will start with data. The fairness of an AI model can be limited by the fairness of the data you feed it. Unfortunately, too often, the data is reflective of the bias that exists in our society. This bias can appear in multiple forms. One form of bias will be the lack of females represented in the data. Another form of bias is where the data has not been appropriately sex dis-aggregated. That is, females are assumed to follow the same distribution and patterns as men.
One such area of research where this issue is particularly troublesome is in medical research. Prior to 1993, when the FDA and NIH mandated the inclusion of women in clinical trials, many medical trials featured no women due to their childbearing potential and complications in retrieving consistent data due to monthly hormonal fluctuations. A 2010 study found that single-sex studies of male mammals in the field of neuroscience outnumbered those of females 5.5 to 1. As a result, in order to account for women in medical trials, results from the male-dominated trials are often extrapolated and women are simply treated as scaled-down men, as explained by Caroline Criado-Perez, author of Invisible Women. This evidently has profound impacts on the health of women. Women are more than 50% more likely to receive an incorrect diagnosis when they are having a heart attack.
How bias can be amplified when implementing a Machine Learning or AI model
Bias in data alone is bad enough, as it can portray incorrect distributions of observed behaviors. However, if you train an AI model on this data, if not done correctly, the model can learn these biased observations and further exacerbate them.
A study assessing digital biomarkers (physiological, psychological, and behavioral indicators) for Parkinson’s disease featured only 18.6% women. Even if you correctly account for gender bias, you are more likely to produce more accurate diagnoses for men than for women due to the larger sample size. However, in the worst-case scenario, if you don’t account for gender at all, you could be misdiagnosing women completely if they exhibit different symptoms to men. Davide Cirillo et al. published an article examining the prevalence of gender bias in AI for healthcare, where it is also suggested that Precision Medicine (as opposed to a one-size-fits-all approach) should be applied.
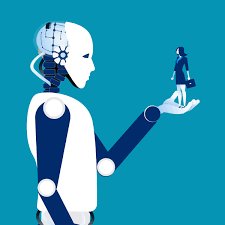
Another such AI model that amplifies gender biases exists in the field of natural language processing (NLP). Let’s say you blindly train a language translation model and ask it to translate the word ‘doctor’ from English into French. Due to historical biases that exist, the model will translate the word into the masculine version, as opposed to the feminine version. This is precisely what happened with google translate.
The NLP technique of word embeddings is also not immune to gender bias. A word embedding represents each word in a piece of text as a vector. A word-embedding model is trained on co-occurrences of words. For example, two words that may have a similar meaning would most likely be located close to each other in the vector space. Furthermore, the distance between such vectors can represent the relationship between words. One such illustrative example given in the paper is:
Man — women ≈ king — queen.
This is innocent enough. The difference between man and woman is similar to the difference between the king and queen. However, it is also the case that
Man — women ≈ computer programmer — homemaker.
Gender bias is also very present in Google search engines. Women are significantly less likely to be shown in online ads for highly paid jobs. 1,000 users were simulated, half male and half female. Male users were shown adverts for jobs paying over $200,000 1,800 times, whereas women were shown those adverts only 300 times.
Algorithm bias can also directly exacerbate the lack of gender representation in tech. An amazon algorithm that was used as a hiring tool penalized women. The algorithm had been trained on historical résumés, which were of course mostly male. The algorithm, therefore, assumed that male candidates were preferable. Even if the gender was explicitly removed from the résumé, there are still features in the résumé that indicates gender, such as being a member of the ‘women’s chess team’, attending an all-female college, or even the language that is used.
Examples like these can further cement the societal gender bias and stereotype that women are not meant to work in tech, or other STEM occupations, making it harder to overcome the current lack of female representation.
The insight women can bring to a team
Many of the issues encountered in the sections above, could have been avoided if more women were present in research and data roles. Kate Crawford, a principal researcher at Microsoft, said that
“Like all technologies before it, artificial intelligence will reflect the values of its creators. So inclusivity matters — from who designs it to who sits on the company boards and which ethical perspectives are included. Otherwise, we risk constructing machine intelligence that mirrors a narrow and privileged vision of society, with its old, familiar biases and stereotypes.”
Women are aware that we are not just a scaled-down version of men. A team that is entirely male, would be less likely to notice the omission of women in their data and would also be less likely to be concerned with the issues that face the other sex. Historically, men have been viewed as the default gender. Introducing more females into data science teams can help combat this.
A particular example of the consequences of this disparity in the world of tech is how Apple iPhones have been designed to fit in the size of a male hand, rather than a smaller female hand. With more women on the team, this would have been much more apparent. A more severe consequence of the lack of consideration of females is that in the design of airbags. Women are 47% more likely to be seriously injured in a crash. Women are again treated as down-scaled men. Car crash test dummies are built to represent the average male. This is not that much of a surprise when you consider the all-male airbag design team. However, even physically speaking, women are not down-scaled versions of men. When thinking of an airbag expanding into my chest, it does not take me long to realize that the existence of breasts may affect their efficacy!
Issues that affect women more than men are also not considered. For example, in the design of a virtual reality game by an all-male team, the issue of sexual harassment, something that most women have to deal on a weekly basis, was not considered. When this game was sent out to a female gamer for review, another (male) gamer proceeded to sexually harass her in the virtual world. Credit should be given to the team who immediately responded and resolved the issue. However, if there were a woman on the team, it would be far more likely that sexual harassment would have been brought up in the design process, given how it is such a regular occurrence in our lives.
The benefit of having a more gender diverse team
This point is not unique to the fields of tech and data. Roughly half of the global population is female. If females are not considered in the design of any product or solution, then you are missing out on half of your potential audience. If the needs and preferences of women are not acknowledged, women may be less likely to buy your product or use your solution, such as a virtual reality game or a smartphone, which would clearly have a negative impact on sales. But, more critically, the lack of consideration or optimization for gender could further inhibit the lives of women relative to men, as demonstrated in AI for healthcare, the design of airbags, or the implementation of hiring algorithms.
Hiring more females into data and AI roles is not just the right thing to do, but it can also benefit your business and improve the fairness of your algorithms. According to Gartner Inc.,
“By 2022, 85% of AI projects will deliver erroneous outcomes due to bias in data, algorithms or the teams responsible for managing them. This is not just a problem for gender inequality — it also undermines the usefulness of AI”.
I would also like to add that gender representation is not the only important form of representation. Many of the examples I gave in this post about a lack of female representation can be repeated for a lack of racial or socioeconomic representation.
Hiring a diverse team is not just about filling quotas, but it can also introduce more perspectives and an improved decision-making process.
In order to correct for gender bias in Data and AI, the approach should be multi-pronged.
- Care should be taken to de-bias data.
- Algorithms should be transparent and tested for bias.
- Companies with Data and AI teams should make more of an effort to ensure that their teams are sufficiently diverse.
If you are interested in learning more about the issues of societal bias in data and algorithms, while supporting the voices of women in the world of Maths, Data, and Tech, I would recommend reading:
- Invisible Women: Exposing Data Bias in a World Designed for Men by Caroline Criado-Perez
- Weapons of Math Destruction: How Big Data Increases Inequality and Threatens Democracy by Cathy O’Neill
- Hello World: Being Human in the Age of the Machine by Hannah Fry